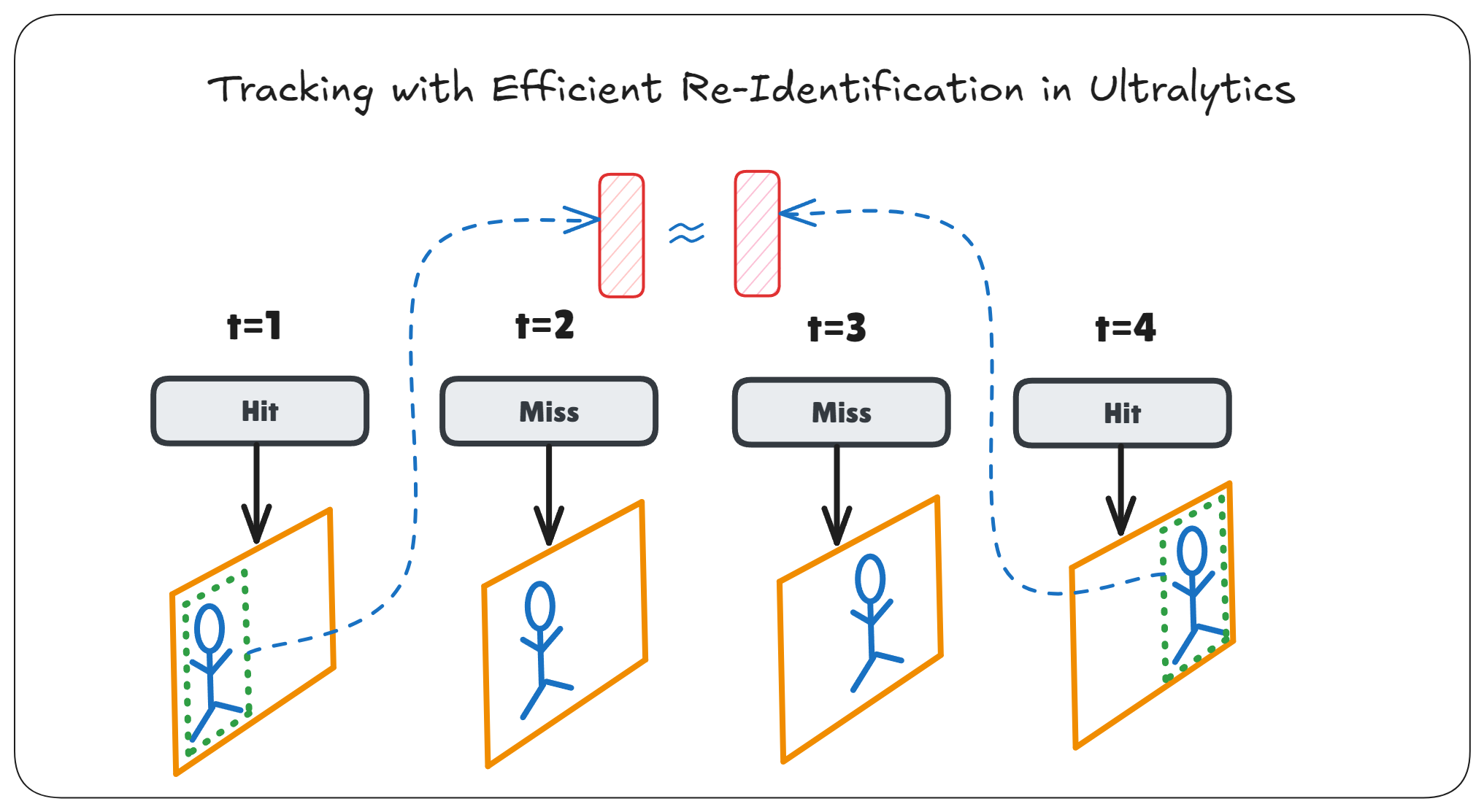
Introduction
Reidentification allows for short-term recovery of lost tracks in tracking. It is usually done by comparing visual similarity between objects using embeddings, which are typically generated by a separate model that processes cropped object images. However, this adds extra latency to the pipeline. We have previously looked at a way to obtain the object-level features from YOLO. These features can also be used for reidentification, removing the need for a separate embedding model. This makes the process more efficient, with practically no impact on latency. In this guide, we will enable tracker reidentification in Ultralytics using these object-level features. The full implementation is available in this Colab notebook.
Update (23/4/2025): The technique shown here has been natively integrated into Ultralytics in v8.3.114
. So you can just use it directly without patching by setting with_reid: True
in botsort.yaml
. You can still read on if you want to learn how it works.
Patching Ultralytics for Re-Identification
The steps for obtaining the object-level features have already been covered in an earlier tutorial. Once you have the pipeline for that ready, you would then need to patch the Ultralytics tracker to use the extracted features for reidentification. The BoTSORT tracker integrated into Ultralytics already has the required methods for reidentification. It just needs to be provided with the features. We begin by modifying the update()
method of BoTSORT to pass the features during the initialization and update of tracklets:
--- a/ultralytics/trackers/byte_tracker.py
+++ b/ultralytics/trackers/byte_tracker.py
@@ -290,9 +290,10 @@ class BYTETracker:
self.kalman_filter = self.get_kalmanfilter()
self.reset_id()
- def update(self, results, img=None):
+ def update(self, results, img=None, feats=None):
"""Updates the tracker with new detections and returns the current list of tracked objects."""
self.frame_id += 1
+ self.img_width = img.shape[1]
activated_stracks = []
refind_stracks = []
lost_stracks = []
@@ -315,8 +316,10 @@ class BYTETracker:
scores_second = scores[inds_second]
cls_keep = cls[remain_inds]
cls_second = cls[inds_second]
+ feats_keep = feats[remain_inds]
+ feats_second = feats[inds_second]
- detections = self.init_track(dets, scores_keep, cls_keep, img)
+ detections = self.init_track(dets, scores_keep, cls_keep, feats_keep)
# Add newly detected tracklets to tracked_stracks
unconfirmed = []
tracked_stracks = [] # type: list[STrack]
@@ -347,7 +350,7 @@ class BYTETracker:
track.re_activate(det, self.frame_id, new_id=False)
refind_stracks.append(track)
# Step 3: Second association, with low score detection boxes association the untrack to the low score detections
- detections_second = self.init_track(dets_second, scores_second, cls_second, img)
+ detections_second = self.init_track(dets_second, scores_second, cls_second, feats_second)
r_tracked_stracks = [strack_pool[i] for i in u_track if strack_pool[i].state == TrackState.Tracked]
# TODO
dists = matching.iou_distance(r_tracked_stracks, detections_second)
We would then also need to patch the on_predict_postprocess_end()
callback in Ultralytics which is called after detection to perform the tracking to pass the feats
that we extracted (refer to earlier tutorial):
--- a/ultralytics/trackers/track.py
+++ b/ultralytics/trackers/track.py
@@ -80,7 +80,7 @@ def on_predict_postprocess_end(predictor: object, persist: bool = False) -> None
det = (predictor.results[i].obb if is_obb else predictor.results[i].boxes).cpu().numpy()
if len(det) == 0:
continue
- tracks = tracker.update(det, im0s[i])
+ tracks = tracker.update(det, result.orig_img, result.feats.cpu().numpy()) # pass feats here
if len(tracks) == 0:
continue
idx = tracks[:, -1].astype(int)
With the features now sent to the tracker, the final step is enabling reidentification. We can do that by patching the BOTSORT
class with a modified version that enables reidentification. BOTSORT can use an encoder to obtain the embeddings using a separate model, but since we are using features that were already extracted, we create a pseudo-encoder that simply return the features:
# Encoder model that's used by BoT-SORT to get embeddings. We use it to simply return the features extracted by YOLO.
class Encoder:
def inference(self, feat, dets):
return feat
# Override the BOTSORT class to use our encoder
class BOTSORTReid(BOTSORT):
def __init__(self, *args, **kwargs):
super().__init__(*args, **kwargs)
self.encoder = Encoder()
self.args.with_reid = True
track.TRACKER_MAP["botsort"] = BOTSORTReid # patch with our class
By default, BoTSORT uses a proximity filter based on IoU to eliminate candidates when matching tracks using the embeddings. I found the IoU-based filter to cause issues when the object moves some distance away due to occlusion. So I patched it with a different proximity filter that uses L2 distance of the centroids instead:
# Create a better proximity mask based on centroid distance
def get_centroid(tlwh):
x, y, w, h = tlwh
return np.array([x + w / 2, y + h / 2])
def get_dists(self, tracks, detections):
"""Calculate distances between tracks and detections using IoU and optionally ReID embeddings."""
dists = matching.iou_distance(tracks, detections)
if self.args.fuse_score:
dists = matching.fuse_score(dists, detections)
if self.args.with_reid and self.encoder is not None:
track_centroids = np.array([get_centroid(track._tlwh) for track in tracks]).reshape(len(tracks), 2)
det_centroids = np.array([get_centroid(det._tlwh) for det in detections]).reshape(len(detections), 2)
# Compute pairwise L2 distances
l2_dists = np.linalg.norm(track_centroids[:, None, :] - det_centroids[None, :, :], axis=2)
l2_dists = l2_dists / self.img_width # Normalize by image width
dists_mask = l2_dists > self.proximity_thresh
emb_dists = matching.embedding_distance(tracks, detections) / 2.0
emb_dists[emb_dists > (1 - self.appearance_thresh)] = 1.0
emb_dists[dists_mask] = 1.0
dists = np.minimum(dists, emb_dists)
return dists
I also increased the track_buffer
in botsort.yaml
to 300
so that it can tolerate occlusion for longer and set the proximity_thresh
and appearance_thresh
to 0.2
and 0.3
respectively based on my tests on what worked best. Increasing the proximity_thresh
allows matches with an object that are farther away, while increasing appearance_thresh
makes the visual similarity more strict. So an appearance_thresh
of 0.8
means the similarity should be greater than 80%, while a proximity_thresh
of 0.3
means the distance between the centroids of the detection and the tracklet in consideration should be less 30% of the image width.
Note that in the official implementation in Ultralytics v8.3.114
, it still uses the IoU-based proximity filter, where it’s defined as the minimum IoU required for the re-identification to be considered valid. So increasing proximity_thresh
in the official implementation makes the matching criteria more strict.
To perform tracking with reidentification, we initialize the model and patch the tracker with our modified methods:
model = YOLO("yolo11n.pt")
embed = model.model.yaml['head'][-1][0] # find the FPN layer indices
embed += [len(model.model.model) - 1] # last layer, i.e. the final output
# Monkey patch method to use the new embed method
model.model._predict_once = MethodType(_predict_once, model.model)
model.track(embed=embed, persist=True) # initialize. embed would make the output also return the outputs from the FPN layers
model.predictor.trackers[0].reset()
# Patch the tracker methods with our updated ones
model.predictor.trackers[0].update = MethodType(update, model.predictor.trackers[0])
model.predictor.trackers[0].get_dists = MethodType(get_dists, model.predictor.trackers[0])
And then run tracking using the following function that first calls the get_result_with_features()
to obtain results
with the extracted feats
and then manually runs the on_predict_postprocess_end()
method to perform tracking in Ultralytics:
def track_with_reid(img):
model.predictor.results = get_result_with_features([img])
model.predictor.run_callbacks("on_predict_postprocess_end") # update tracks
return model.predictor.results
Re-Identification In Action
When comparing the output with and without reidentification, we observe virtually no drop in FPS. And we also see that tracker is able to recover the tracks much better after occlusion.
Conclusion
In this guide, we incorporated the extracted features from YOLO for reidentification with almost zero hit to inference FPS. You will probably need to play with the tracker parameters, particularly proximity_thresh
and appearance_thresh
, to get the best trade-off between correct reidentification and incorrect ID swaps.
Thanks for reading.